Cureline’s Artificial Intelligence-based Hit Identification
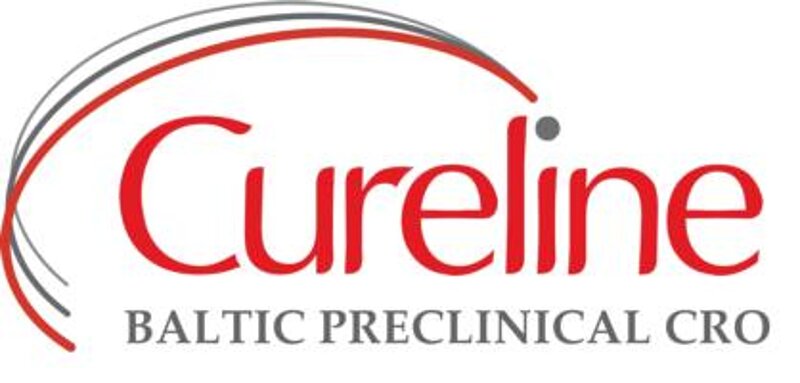
Cureline uses a deep learning-based technology that predicts molecule properties with unparalleled accuracy, rivaling experimental precision. This technology led to the discovery of new preclinical leads at negligible costs. Its versatility across therapeutic areas has been validated by independent laboratories in virology and oncology.
Cureline’s services span the entire spectrum of preclinical drug development, from Hit Discovery to Lead Optimization. This encompasses virtual High Throughput Screening (HTS) for hit identification, ADMET property predictions, Off-target effect predictions, and Hit Analog virtual screening. Their system enables the virtual screening of a billion compounds per day.
Case Study: Discovery of Novel Antivirals Against COVID-19
Working with a virlogy lab in Denmark, Cureline was able to discover new antivirals against SARS-CoV-2.
By training neural networks on various experiments around SARS-CoV-2 inhibition and cellular toxicity, Cureline was able to select six never-before-tested compounds against COVID-19. Their AI’s predictions of new anti-SARS-CoV-2 drug candidates were confirmed in vitro with high success rates in multiple cellular assays. View the experimental activity of Cureline’s predicted SARS-CoV-2 inhibitors in cell cultures below.
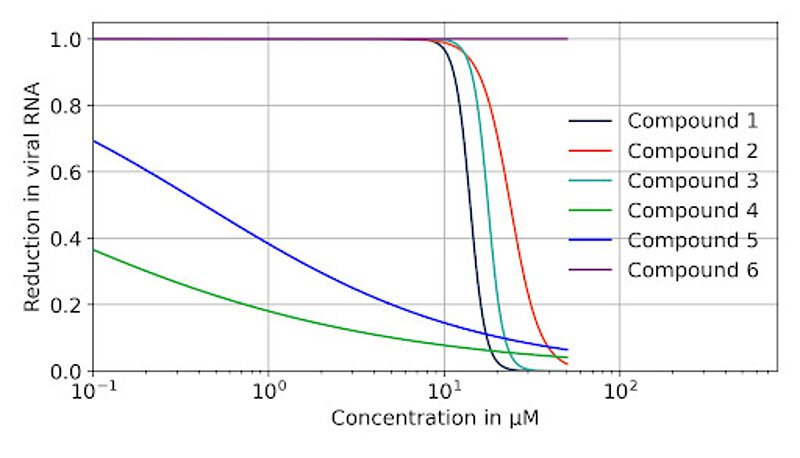
Explore detailed descriptions and more case studies to see Cureline’s capabilities in action here.
By combining hit discovery with lead optimization in a single powerful step, the technology achieves a leap in cost-effectiveness.
Deep Learning-Based QSAR
Quantitative Structure-Activity Relationship powered by novel deep learning-based graph neural network architectures enable Cureline to train on their proprietary database of HTS data. This database encompasses about 260M data points, 2.5M molecules, and 2000 properties.
Cureline’s technology harnesses the power of modern deep learning approaches within innovative neural network architectures understanding chemistry. One of the milestones achieved was to learn from very scarce data and reach experimental reproducibility in terms of property prediction accuracy.
Unique features of the system
- End-to-end training: learn to relate raw data to raw predictions
- Massive multi-tasking: thousands of properties simultaneously predicted
- Structured covariant neural networks (NN): tailored to biochemistry
- NN complexity: smarter algorithms with greater predictive abilities.
Cureline’s unique selling points
- Proven: tested by independent laboratories
- Accurate: unprecedented prediction precision, on par with experiments
- Fast: 10k molecules per second
- Versatile: applicable in any therapeutic area
- Multiparametric: learns from all properties simultaneously
Download Cureline’s whitepaper to explore the specifics of our AI-driven approach.