VAIDR: The Visual AI for Drug Research
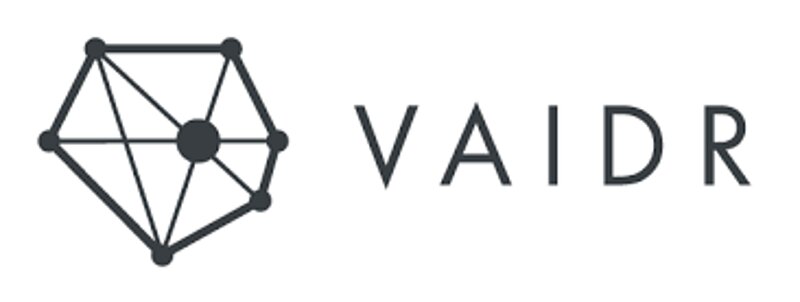
VAIDR is TRI Thinking Research Instruments’ solution for cell image analysis. VAIDR not only works on fluorescently labelled microscopic images but label-free images as well. Read below to learn how VAIDR is used in Cell Segmentation.
VAIDR is used to avoid having to adapt multiple parameters to remove evident flaws in segmentation. The goal was to create a workflow that produces algorithms that can learn to answer questions relevant to specific types of data sets. VAIDR eliinates the need for highly specific skills or experience in manually tuning parameters in scripting languages or graphical user interfaces, therefore saving time and facilitating the segementation process.
Cell Segmentation Using VAIDR
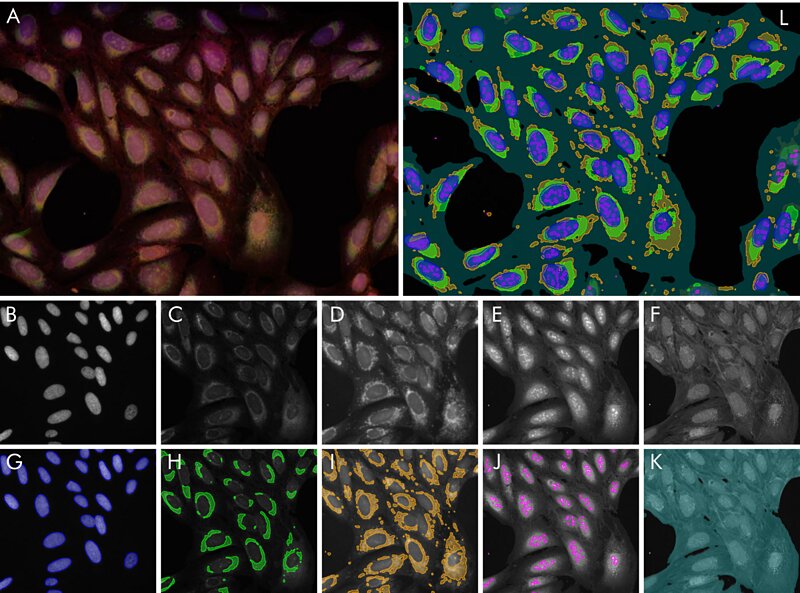
overlaid on raw channels (G – K) and overlay of all segmentations (L).
In this cell segmentation example, TRI trained five algorithms to solve segmentation tasks on a multi-channel fluorescence dataset, published by the Broad Institute1. From TRI’s Cell Segmentation application note2 , “nucleus segmentation is performed after labeling based on the nuclear stain Hoechst. The results were already near perfect after a single labeled image. Nucleoli, endoplasmic reticulum, mitochondria are detected by their own algorithms, respectively. No algorithm needed more than one fully labeled image and at most one or two images which were partially corrected. In total, the effort of labeling by the user amounted to less than one hour. The outcome are five segmentation methods which can be immediately used to robustly generate quantitative results for a plethora of biological questions: Size, shape and number of nuclei and nucleoli. Relative areas and intensities of organelles like mitochondria, or total confluency. With a little post-processing, the number of useful output parameters can be increased drastically: How strong is the striation in the non-nuclear regions of the cell due to the actin stain? What is the overlap between the mitochondria region and the ER-region? And so on.”
VAIDR makes this possible as scientists simply need to provide the segmented images for training. During training, the AI is “rewarded” if it correctly predicts the traning labels, leading to self-improvement of the system while continuing its processing of the user-supplied set of images and labels. The AI then develops these skills automatically. The AI continues to develop different “notions” into its skillset: if it recognizes that a pattern is more relevant, it will encode them and will be able to use them in different applicatinos.
Learn More
To read more about VAIDR’s cell segmentation example and other application notes, click the button below.
References
- Gustafsdottir SM, Ljosa V, Sokolnicki KL, Anthony Wilson J, Walpita D, Kemp MM, et al. (2013) Multiplex Cytological Profiling Assay to Measure Diverse Cellular States. PLoS ONE 8(12): e80999. doi.org/10.1371/journal.pone.0080999.
- Cell Segmentation by User-Trainable AI