3 Bottlenecks Keeping Big Data From Big Results
This is Part 2 in a three-part series on Big Data in the pharma and biotech industries.
(Check out Part 1 and Part 3).
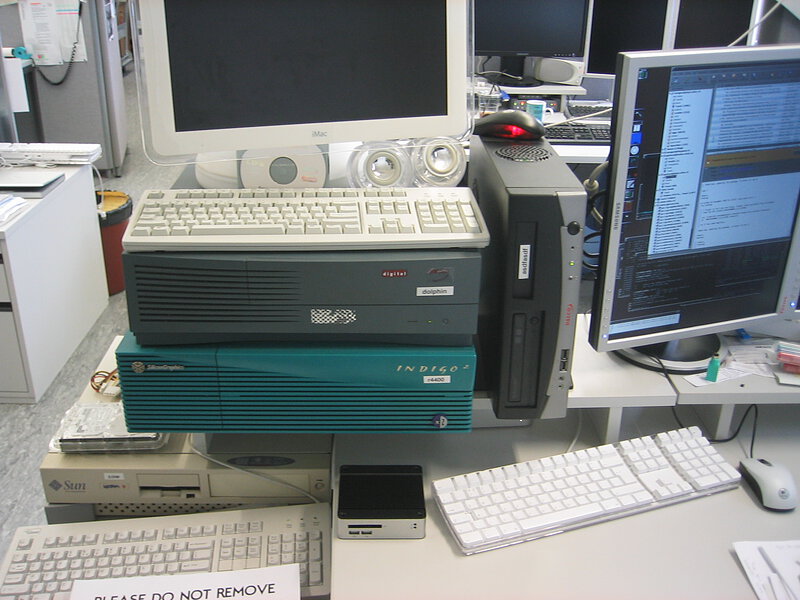
Recent medical technology advances — in high-content screening, next-gen DNA sequencing, wireless health monitoring, just to name a few — are a boon for medical research and healthcare. At least they hold great promise for accelerating disease prevention, diagnosis, and treatment. In the meantime, these mounting piles of hits and heartbeats have researchers drowning in datasets so large and complex that they often can’t be effectively stored or analyzed. Instead, data is underutilized or lost altogether.
Biological Big Data projects often choke up at three main bottlenecks:
1. Complexity
Compared to other fields, biological systems pose an additional hurdle — sheer complexity.1 The number of unknowns and potential variables in living systems make it hard to combine and fully complete datasets enough to make them useful. For example, you can sequence a genome. But then there’s expression status. Which genes are on or off? How are they regulated? Even tougher — how do sequence and expression correlate with clinical symptoms? What about all the meta-data that goes with each human clinical sample — gender, age, geographical location, and other health information? There’s no standard for the type of information that needs to be collected and how to record it. As a result, datasets from different sources can rarely be combined or compared.
2. Shortage of computing power
Standard desktop computers and software — the kind found in most biomedical research labs — can’t store and process the amount of data being generated. In biological research, funding is more readily available for generating data than for analyzing it. In some cases, biologists have teamed up with supercomputer centers, but they are usually geared toward physics and engineering, rather than biological systems. To share data, researchers still often send hard drives by snail mail. Stranger still, since the cost of gene sequencing has fallen faster than the cost of data storage and shipping, it can actually be cheaper to send and store biological samples for sequencing than it is to send the data.
3. Lack of accessibility to Big Data
If biological Big Data are to be useful, they have to be accessible to more than one group. The more minds contributing, mining, and analyzing, the more Big Data could benefit science. Yet often this isn’t the case. Academia’s “silo” culture, intellectual property and copyright issues (both real and perceived), fear of being “scooped,” stiff competition for grant funding, and lack of awareness of online communication tools are major hurdles standing in the way of Open Science — the movement toward freely sharing research methodology, raw data, and results online, as well as encouraging open participation from scientists and non-scientists of all backgrounds.
What do YOU think is slowing the Big Data revolution in biomedical research and drug development?
Continue with Part 3 in this series: 3 Ways to Avoid Drowning in Your Big Data.
References
- Singer, E. Biology’s Big Problem: There’s Too Much Data to Handle. Wired. October 11, 2013.